Brent van Herk
Create Your First Project
Start adding your projects to your portfolio. Click on "Manage Projects" to get started
Early Stroke Detection Device
Date
2023
Project type
Master BME Course
Each year, about 15 million people worldwide suffer a stroke. From these, about one-third die and another third is permanently disabled, which places a burden on family and community. Nowadays, no device for early detection of a stroke is available. Therefore, a stroke is only detected after onset of the symptoms such as numbness, trouble speaking, trouble seeing, trouble walking or severe headache. Then immediate treatment is needed to prevent irreversible brain damage. However, as the numbers show, treatment often comes too late. People suffering a stroke will die or be disabled and only a small number of people are getting treatment in time. Therefore, early detection of a stroke is necessary to improve the prognosis of a stroke.
This device uses a 30 by 20 mm ultrasound probe to monitor the plaque buildup in the carotid artery. It has a thickness of 3 mm and a weight of 40 grams. It has two probes that can be placed on both sides of the neck. The transducer has a density of 400 elements cm^2 and is via an elastic couplant connected to the skin. This couplant is made of a hydrogel that is composed of a polymer network of chitosan-polyacrylamide and water. This network is surrounded by polyurethane to provide a connection to the skin and to prevent the probe from deforming the skin. The highly detailed image gets sent to the processing unit.
On the processing unit, the images are pre-processed to enhance their quality, remove noise, and optimize the data for analysis. Ideally, only the artery and the plaque buildup should be left over after this step, to reduce the processing time of future steps. From these processed images, the plaque buildup can be calculated.
If there is plaque buildup happening inside the artery, the ultrasound images show the buildup as a higher white value than the surrounding tissue. The device will use random forest analysis to classify and predict the outcomes. This is a machine-learning technique that can recognize image characteristics such as texture, brightness, and spatial patterns. This model has already been trained using 159 images with carotid plaque buildup and 202 normal images and was able to predict new images very accurately with an accuracy of 91.41% and a precision of 94.29%. Following this machine learning technique, the device uses a threshold mechanism. When the degree of plaque buildup exceeds a threshold of 70%, the device triggers an electrical signal that is converted to an alarm. This alarm notifies the patient that there is potentially a stroke going to happen in a few hours.
The probes and the processing unit work together to alert the patient that their vascular systems need medical attention, and the device allows for an early intervention.
The pods are connected to the processing unit. This unit contains a clip that allows the user to attach the unit to the patient's clothes. The unit reads the data that de pods measure and processes this data in real time. The processing unit contains a button to turn the device on or off and start the measurements. A small display is included to provide the user with information about the status of the device. Information such as battery life, remaining measuring time and potential warnings can be shown on the display. The processing unit can be connected to an app via Bluetooth, to view detailed information about the measurements, send the data to the healthcare professionals and give health risk warnings.

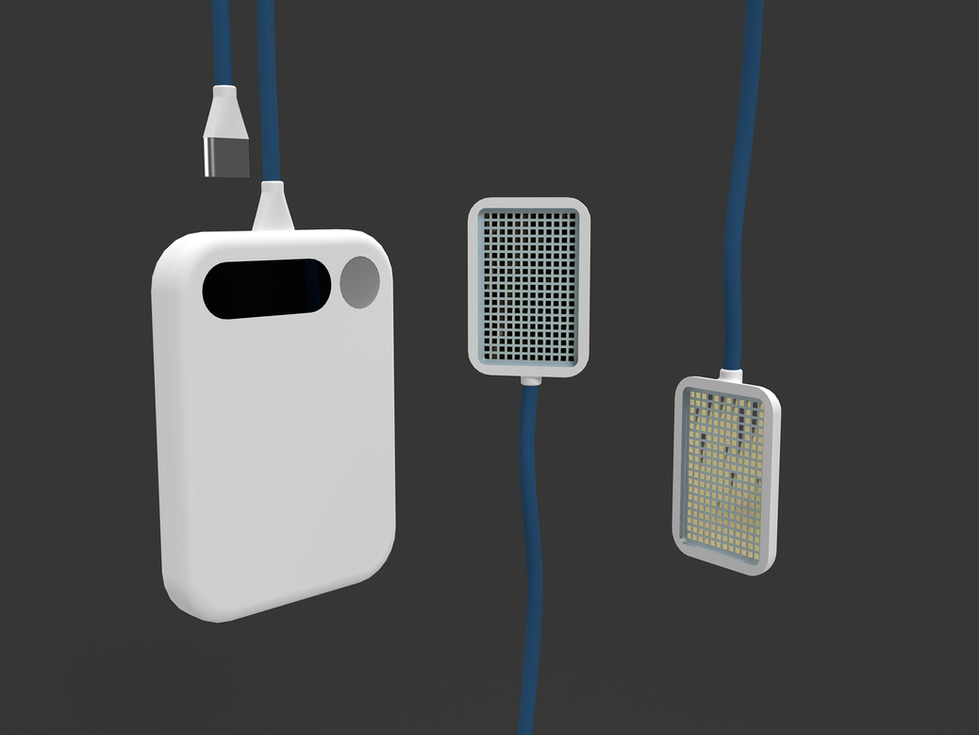